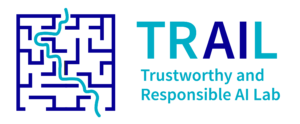
We are pleased to invite you to the 4th seminar of the Trustworthy and Responsible AI Lab, an AXA-Sorbonne University joint research lab.
Human-Centered AI: How Can We Support End-Users to Interact with AI?
Katrien Verbert, Professor at KU Leuven
Visual Explanations for AI Decisions: Fostering Trust in AI through Transparency and Control
Jeroen Ooge, PhD Candidate at at KU Leuven
Friday April 7th 2023, 15:00 – 16:30 CET
On-site
On registration, within the limit of available seats
Sorbonne University – Faculté des Sciences
Salle de conférences, SCAI, bâtiment Esclangon, 1er étage
Campus Pierre et Marie Curie, Sorbonne Université
4 place Jussieu, 75005 Paris
Or Online
MS Teams link upon registration
> Registration <
Agenda:
Human-Centered AI: How Can We Support End-Users to Interact with AI?
Katrien Verbert, Professor at the Augment research group of the Computer Science Department of KU Leuven
Despite the long history of work on explanations in the Machine Learning, AI and Recommender Systems literature, current efforts face unprecedented difficulties: contemporary models are more complex and less interpretable than ever. As such models are used in many day-to-day applications, justifying their decisions for non-expert users with little or no technical knowledge will only become more crucial. Although several explanation methods have been proposed, little work has been done to evaluate whether the proposed methods indeed enhance human interpretability. Many existing methods also require significant expertise and are static. Several researchers have voiced the need for interaction with explanations as a core requirement to support understanding. In this talk, I will present our work on explanation methods that are tailored to the needs of non-expert users in AI. In addition, I will present the results of several user studies that investigate how such explanations interact with different personal characteristics, such as expertise, need for cognition and visual working memory.
Katrien Verbert is Professor at the Augment research group of the Computer Science Department of KU Leuven. She obtained a doctoral degree in Computer Science in 2008 at KU Leuven, Belgium. She was a postdoctoral researcher of the Research Foundation – Flanders (FWO) at KU Leuven. She was an Assistant Professor at TU Eindhoven, the Netherlands (2013 –2014) and Vrije Universiteit Brussel, Belgium (2014 – 2015). Her research interests include visualisation techniques, recommender systems, explainable AI, and visual analytics. She has been involved in several European and Flemish projects on these topics, including the EU ROLE, STELLAR, STELA, ABLE, LALA, PERSFO, Smart Tags and BigDataGrapes projects. She is also involved in the organisation of several conferences and workshops (general co-chair IUI 2021, program chair LAK 2020, general chair EC-TEL 2017, program chair EC-TEL 2016, workshop chair EDM 2015, program chair LAK 2013 and program co-chair of the EdRecSys, VISLA and XLA workshop series, DC chair IUI 2017, DC chair LAK 2019).
Visual explanations for AI decisions: Fostering trust in AI through transparency and control
Jeroen Ooge, PhD Candidate in computer science at KU Leuven
Automated systems increasingly support decision-making with AI. While such automation often improves working efficiency, it also raises questions about the origin and validity of model outcomes. Explaining model outcomes is not trivial: AI models are black boxes to people unfamiliar with AI. A promising solution to realise explainable AI (XAI) is visualisation. Through interactive visualisations, people can better understand models’ behaviour and reasoning process, which helps them contextualise model outcomes. Important here is that different people and different contexts require different solutions. Thus, human-centred XAI methods are essential. In this talk, Jeroen will cover his XAI work on transparency and control, applied in healthcare and education. He will demonstrate some of the many visual interfaces he designed, and also present the user studies he conducted to study their impact on people’s behaviours, for example, their trust in AI decisions.
Jeroen Ooge holds two Masters of Science (fundamental mathematics and applied informatics) and now finalises a PhD in computer science at KU Leuven in Belgium. His research focuses on explainable AI. In particular, Jeroen investigates how visualisations can help people to better understand AI models, calibrate their trust in these models, and steer model outcomes with domain expertise. He has studied and designed numerous interactive visualisations tailored to a specific target audience and application context.
About TRAIL, the joint research lab
The Trustworthy and Responsible Artificial Intelligence Lab (TRAIL) is a Sorbonne University and AXA joint research lab. Its aim is to study and address challenges linked to a generalized use of AI systems, in particular the explainability, fairness, robustness, governance and regulation of AI. The lab is composed of researchers from Sorbonne University and AXA. It is based in the Pierre & Marie Curie Campus at the Faculté des Sciences de Sorbonne University, inside the LIP6, a Computer Science lab from Sorbonne University and the CNRS (French National Center for Scientific Research) in Paris.