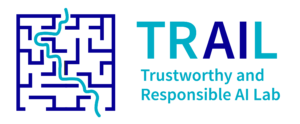
We are pleased to invite you to the 8th seminar of the Trustworthy and Responsible AI Lab, an AXA-Sorbonne University joint research lab.
For this seminar, we are excited to introduce a new format where we invite doctoral students specializing in responsible AI to share their research.
PhD Panorama on Responsible AI:
Three Talks on Regulation, Ethics, and Explainability
Mélanie Gornet, PhD Student at Télécom Paris
Milan Bhan, PhD Student at Sorbonne University
Thomas Souverain, PhD Student at ENS Ulm
Thursday November 16th 2023, 16:00 – 18:00 CET
Sorbonne University – Faculté des Sciences
Campus Pierre et Marie Curie
4 place Jussieu, 75005 Paris
Tower 24 → 4th floor → Corridor 24-25 → Room #405 (at LIP6 lab)
See Campus Map
Attend On-Site
On registration, within the limit of available seats
Attend Online
Link to the Microsoft Teams event upon registration
The European approach to regulating AI through technical standards, Mélanie Gornet
European institutions are currently finalizing the AI Act, a new regulation on artificial intelligence. The AI Act will require manufacturers of high-risk AI systems to affix a European Conformity (CE) mark on their products to show compliance with the AI Act and permit AI products to circulate freely in Europe. The issuance of a CE mark will depend on compliance with harmonized technical standards (HSs), developed by European standardization organizations. HSs and CE marking are long-established regulatory techniques in the EU to deal with product safety. To date, however, they have never been used to attest to compliance with fundamental rights, and yet part of the goal of the AI Act is to ensure that AI systems respect these rights.
What is the role of HSs and CE marking in the AI Act and how does it differ from other European legislation? What problems are posed by the use of HSs and CE marking to assess the protection of fundamental rights and can they be avoided?Mélanie Gornet is a PhD student at Télécom Paris, Institut Polytechnique de Paris.
She focuses on regulation of artificial intelligence that encompasses social, legal and technical aspects. Her current projects focus on the standardisation and operational implementation of ethical criteria for AI systems, such as explainability and fairness. She is particularly interested in the compliance of AI systems in the context of the proposed European AI Act regulation.
She previously studied at SciencesPo Paris and ISAE-SUPAERO, specializing in AI and data science, and accompanied the working group on facial and behavioural recognition of the Comité National Pilote d’Ethique du Numérique (CNPEN).
Generating textual counterfactual explanations, Milan Bhan
Counterfactual examples explain a prediction by highlighting changes of instance that flip the outcome of a classifier. This work proposes TIGTEC, an efficient and modular method for generating sparse, plausible and diverse counterfactual explanations for textual data. TIGTEC is a text editing heuristic that targets and modifies words with high contribution using local feature importance. A new attention based local feature importance is proposed. Counterfactual candidates are generated and assessed with a cost function integrating semantic distance, while the solution space is efficiently explored in a beam search fashion. The conducted experiments show the relevance of TIGTEC in terms of success rate, sparsity, diversity and plausibility. This method can be used in both model-specific or model-agnostic way, which makes it very convenient for generating counterfactual explanations.
Even under sparsity constraint, counterfactual generation can lead to numerous changes from the initial text, making the explanation hard to understand. We propose Counterfactual Feature Importance, a method to make non-sparse counterfactual explanations more intelligible. Counterfactual Feature Importance assesses token change importance between an instance to explain and its counterfactual example. We develop two ways of computing Counterfactual Feature Importance, respectively based on classifier gradient computation and TIGTEC loss evolution during counterfactual search. Then we design a global version of Counterfactual Feature Importance, providing information about semantic fields globally impacting classifier predictions. Counterfactual Feature Importance enables to focus on impacting parts of counterfactual explanations, making counterfactual explanations involving numerous changes more understandable.Milan Bhan is a PhD student at Sorbonne University, in the LIP6 lab, and senior consultant at Ekimetrics.
His work focuses mainly on interpretability and natural language processing. He has developed a method for generating sparse textual counterfactual examples.
From Explainability to Fairness in AI – Insights of a PhD in Philosophy, Thomas Souverain
Recent Machine Learning (ML) models are commonly considered as “black boxes”, mainly due to their ability to learn statistical patterns from data rather than human rules. Research in philosophy aims to take a step back on this new kind of Artificial Intelligence (AI), in the general sense of attempts to delegate intellective functions to automated computing systems. What is the impact of these opaque models on human understanding and ethical use?
We present here our PhD approach, which digs into the opacity of AI on both sides of fairness and explainability, applied to the cases of loan lending and job offers. The first axis investigates the convergence between the techniques introduced for fairer AI and humans’ ideas of justice. The second one highlights the gap between explainable AI (xAI) techniques and their relevance as perceived by users, putting forward epistemological criteria to complement xAI and shape a full explanation. As explainability and fairness only represent a part of AI Ethics, we finally broaden the pragmatic view on other principles, required to accommodate ML for humans.
Thomas Souverain is currently finishing a Philosophy PhD on AI Ethics at ENS Ulm (2020-2024), on the topic: “Can we Explain AI? Technical Solutions and Ethical Issues in algorithmic Loan Lending and Job Offering”.
To that end, the PhD consists of a CIFRE partnership between the ENS Ulm (Institut Jean Nicod, Paris Sciences et Lettres University) and companies providing Thomas with programming skills and field expertise. Since 2020, Thomas studies AI ethical challenges on applied cases, namely loan lending (DreamQuark) and job offering (Pôle Emploi DSI).
On this rich material from data-science, in particular to correct AI models for fairness purposes or to shape them to be better understood, his advisor Paul Egré (ENS Ulm, IJN, PSL) helps him determine to what extent algorithmic fairness is tied with ideas of justice, and explainable AI techniques can be translated into human categories for understanding.
Beside his speciality on explainability and fairness, Thomas spends time to make AI ethics more familiar and implementable. He teaches students in computer science AI ethics (Paris 13 University master’s degree). During his research stays in Oxford and Stanford, he organized meetings between experts in the law, social sciences, and computer science to bridge the gaps between institutions and disciplines. He also leads since 2021 a working group of AI ethicists and experts (La Poste, Pôle Emploi, Gendarmerie…). In that group of Hub France IA, he develops and shares good practices to anticipate the trust requirements of the European AI Act.